Translate this page into:
Exploring chat generated pre-trained transformer-3 ability to interpret MRI knee images and generate reports
*Corresponding author: Rajesh Botchu, Department of Musculoskeletal Radiology, Royal Orthopedic Hospital, Birmingham, United Kingdom. drrajeshb@gmail.com
-
Received: ,
Accepted: ,
How to cite this article: Saran S, Shirodkar K, Ariyaratne S, Iyengar KK, Jenko N, Durgaprasad B, et al. Exploring chat generated pre-trained transformer-3 ability to interpret MRI knee images and generate reports. J Arthrosc Surg Sports Med. 2024;5:75-80. doi: 10.25259/JASSM_16_2024
Abstract
Objectives:
The study’s objective was to determine if Chat Generated Pre-Trained Transformer-3 (ChatGPT)-4V can interpret magnetic resonance imaging (MRI) knees and generate preliminary reports based on images and clinical history provided by the radiologist.
Materials and Methods:
This cross-sectional observational study involved selecting 10 MRI knees with representative imaging findings from the institution’s radiology reporting database. Key MRI images were then input into the ChatGPT-4V model, which was queried with four questions: (i) What does the image show?; (ii) What is the sequence?; (iii) What is the key finding?; and, (iv) Finally, the model generated a report based on the provided clinical history and key finding. Responses from ChatGPT-4 were documented and independently evaluated by two musculoskeletal radiologists through Likert scoring.
Results:
The mean scores for various questions in the assessment were as follows: 2 for “What does the image show?,” 2.10 for “What is the sequence?,” 1.15 for “What is the key finding?,” and the highest mean score of 4.10 for the command “Write a report of MRI of the…” Radiologists consistently gave mean scores ranging from 2.0 to 2.5 per case, with no significant differences observed between different cases (P > 0.05). The interclass correlation coefficient between the two raters was 0.92 (95% Confidence interval: 0.85–0.96).
Conclusion:
ChatGPT-4V excelled in generating reports based on user-fed clinical information and key findings, with a mean score of 4.10 (good to excellent proficiency). However, its performance in interpreting medical images was subpar, scoring ≤2.10. ChatGPT-4V, as of now, cannot interpret medical images accurately and generate reports.
Keywords
Artificial intelligence
ChatGPT-4
Radiology reporting
Magnetic resonance imaging
Knee
Language models
INTRODUCTION
As technology advances, artificial intelligence (AI) has become one of the most extensively researched fields. In 1950, Alan Turing proposed the Turing test to assess whether a machine can achieve human-level intelligence.[1] The term “AI” was subsequently introduced in 1955 during a 2-month workshop led by McCarthy et al.[2]
Chat Generated Pre-Trained Transformer-3 (ChatGPT-3), an OpenAI model, is a significant contributor to AI-generated content, evolving from GPT-1 in 2018 to its current form in November 2022. Designed as a large language model, ChatGPT-3 serves applications in clinical decision-making and education, generating text-based responses using natural language. Its advantage lies in its ability to respond to multiple languages. A more recent version, GPT-4, introduced in March 2023, is not yet publicly available for free. GPT-4 utilizes supervised and unsupervised learning methods, incorporating vast Internet data and reinforcement learning with human feedback. In health-care scenarios, ChatGPT technology could assist patients in addressing concerns when communicating with doctors. GPT-4, with 1 trillion parameters, surpasses GPT-3, which is trained on 175 billion parameters.[3-5]
ChatGPT holds significant potential in contributing to radiology reporting by aiding radiologists and healthcare professionals in the creation of narrative reports, responding to inquiries, and enhancing communication.[6,7] The utilization of ChatGPT has previously been investigated in the field of oral and maxillofacial radiology, focusing on tasks such as report generation through the identification of radiographic anatomical landmarks. This exploration included learning about the characteristics of oral and maxillofacial pathologies and their corresponding radiographic features.[8] Nevertheless, the application of ChatGPT in the context of composing and structuring reports for magnetic resonance imaging (MRI) of the knee joint remains unexplored.
In this study, we have hypothesized that ChatGPT can help radiologists interpret MRI knees and generate preliminary reports based on images and clinical history provided by the radiologist. As of our latest knowledge update, we are unaware of any published studies specifically examining the role of ChatGPT or similar language models in generating MRI knee reports in the scientific literature.
MATERIALS AND METHODS
Study design
This cross-sectional observational study was conducted in the months of November and December 2023.
Aims and objectives
The study’s objective was to determine if ChatGPT-4V can interpret MRI knees and generate preliminary reports based on images, clinical history and key findings provided by the radiologist.
Inclusion and exclusion criteria
Ten MRI knees with representative imaging findings of different diseases were selected from the institute’s radiology reporting database. Complicated and post-operative cases were excluded.
Data collection
Key MRI images were uploaded on the ChatGPT-4V model in portable network graphics format, and the following questions were asked:
What does the image show?
What is the sequence?
What is the key finding?
Finally, the ChatGPT-4V model was asked to generate a report based on the clinical history provided and key findings, for example: Write a report of MRI of the right knee of a 30-year-old with an ACL tear.
The replies from the ChatGPT-4V model were documented and evaluated by two musculoskeletal radiologists [Table 1]. The evaluation was conducted using a Likert scale ranging from 1 to 5 (1 = Poor, 2 = Fair, 3 = Average, 4 = Good, 5 = Excellent) for the correctness of the information yielded. Evaluations were conducted independently by radiologists to address potential biases, and blinding procedures were put in place to guarantee impartial assessments.
ChatGPT report | Musculoskeletal radiologist | |
---|---|---|
![]() |
What does the image show? ChatGPT The image appears to be a medical scan, specifically an MRI, of a human knee joint. It shows the bones of the knee, including the femur, tibia, and patella, as well as the surrounding soft-tissue structures such as ligaments, meniscus, and possibly cartilage. These types of images are used in musculoskeletal radiology to diagnose conditions such as ligament tears, meniscal injuries, and degenerative changes like arthritis. User What’s the sequence ChatGPT The image provided is a single slice from a sequence of MRI images. MRI sequences are different types of scans that highlight various tissues based on their physical and biochemical properties. Common MRI sequences include T1-weighted, T2-weighted, proton density, STIR, and GRE, among others. The image appears to show high contrast in the fluid, suggesting it could be a T2-weighted sequence, which is good for visualizing fluid and distinguishing it from other soft-tissue structures because fluid appears bright on T2-weighted images. However, without more context or the ability to compare it with other sequences, this is a general assumption. In a full MRI study, several sequences would be captured to provide a comprehensive view of the anatomy and any pathology. User What are the findings on the MRI image ChatGPT As an AI, I’m not able to provide medical diagnoses or interpret clinical images. The image you’ve uploaded appears to be an MRI scan of the knee, and while I can describe its features, such as showing the bones, soft tissues, and potential fluid indicated by brighter areas, any interpretation of specific medical findings should be performed by a qualified medical professional, such as a radiologist. They can provide a detailed assessment, taking into account clinical history, symptoms, and other diagnostic tests to offer accurate medical findings. User Write a report of MRI of the right knee of 30-year-old male with medial meniscal tear ChatGPT Certainly, here’s a sample format for an MRI report of the right knee indicating a medial meniscal tear for a 30-year-old male: Patient: (Patient Name or ID) Age: 30 years Sex: Male Date of Examination: (Date of MRI Scan) Referring Physician: (Doctor’s Name) Type of Study: MRI of the Right Knee Clinical History: Complaints of knee pain and swelling; suspected meniscal injury. Technique: Multiplanar, multi-sequential MRI imaging of the right knee was performed without the use of IV contrast. Findings: Ligaments: The ACL, PCL, and LCL appear intact. No evidence of tear or significant sprain. Menisci: There is increased signal intensity on T2-weighted images within the posterior horn of the medial meniscus extending to the inferior articular surface, consistent with a meniscal tear. No displaced meniscal fragment is noted. Articular Cartilage: The articular cartilage of the medial and lateral compartments is maintained with no significant chondral loss or defect. Bones: No acute osseous injury identified. No evidence of significant marrow edema. Joint Effusion: Mild joint effusion is present. Synovium: No evidence of synovial proliferation. Soft Tissues: The quadriceps and patellar tendons are intact. No soft-tissue masses. Impression: MRI findings suggestive of a tear in the posterior horn of the medial meniscus of the right knee. Mild joint effusion. No acute osseous injury or significant ligamentous abnormality identified. Recommendations: Clinical correlation is recommended. Orthopedic consultation may be warranted for potential management options including, but not limited to, physical therapy, possible meniscectomy, or meniscal repair depending on the patient’s symptoms and clinical goals. Please note that this is a sample report for illustrative purposes only. Actual medical reporting should be done by a qualified radiologist. The findings and recommendations would be tailored to the individual patient’s scans and clinical situation. |
What does the image show? PDFS sagittal image of knee What’s the sequences? PDFS sagittal What are the findings on the MRI images? PDFS sagittal image shows an horizontal oblique cleavage tear of posterior horn of medial meniscus. User Write a report of MRI of the right knee of 30-year-old male with medial meniscal tear MR of the right knee Clinical history-medial knee pain. Technique-PDFS axial, coronal and sag, PD coronal FINDINGS: There is horizontal oblique cleavage tear of body and posterior horn of medial meniscus. ACL, PCL, MCL, LCL, lateral meniscus, iliotibial band, popliteus, posterolateral corner, and extensor mechanism are intact. Type 1 patella which is normally located in the trochlear groove. Articular cartilage is relatively preserved. No joint effusion. No Baker's cyst. Normal signal is noted in the Hoffa’s fat pad, suprapatellar and pre-femoral fat pad. Normal marrow signal is noted in the bones. IMPRESSION Medial meniscal tear. |
ChatGPT: Chat generated pre-trained transformer, MRI: Magnetic resonance imaging, STIR: Short tau inversion recovery, GRE: Gradient recalled echo, PDFS: Proton density fat-saturated, PD: Proton density, sag: sagittal, ACL: Anterior cruciate ligament, PCL: Posterior cruciate ligament, LCL: Lateral cruciate ligament, MCL: Medial cruciate ligament, IV: Intra venous.
Statistical analysis
The results of the retrieved queries were documented in a Microsoft Excel sheet (Microsoft Corporation, Redmond, WA); the statistical analysis primarily focused on descriptive statistics, which summarized the Likert Scale ratings given by the radiologists for each parameter. The results were presented in terms of mean values to capture central tendencies and consensus among evaluators. The interclass correlation coefficient was used to measure agreement between the two raters.
RESULTS
Ten MRI knees with representative imaging findings of different diseases were selected from the institute’s radiology reporting database. Key images that were uploaded on the ChatGPT-4 model are compiled in Figure 1 and Table 1 shows report of one case generated by ChatGPT and the Musculoskeletal Radiologist.
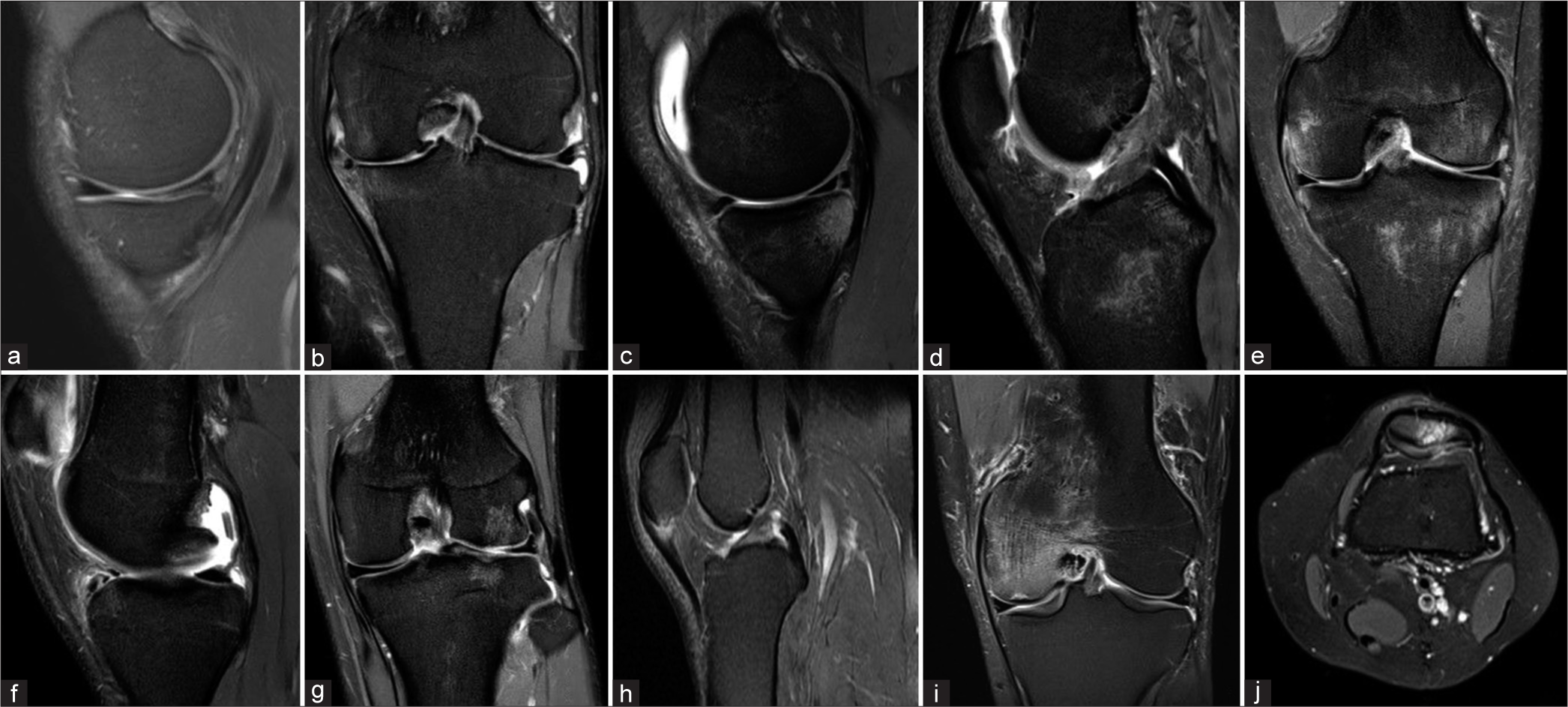
- Key images that were uploaded on the ChatGPT4V model. (a) A 30-year-old male with a medial meniscal tear, (b) a 40-year-old male with severe medial tibiofemoral degenerative change and complex medial meniscal tear, (c) meniscal tear (RAMP lesion) in a 33-year-old male with osseous edema of the posterior part of the medial tibial plateau, (d) a 20-year-old female with an anterior cruciate ligament tear, (e) a 30-year-old male with grade 2 sprain of the meniscofemoral ligament with mild osseous edema of the medial femoral condyle, (f) a 40-year-old male with 10 mm chondral loose body in the posterior recess, (g) a 28-year-old male with a 10 mm chondral defect of lateral femoral condyle with subchondral osseous edema, (h) a 30-year-old male with patellar tendinopathy at the level of the lower pole of patella, (i) a 60-year-old male with subchondral insufficiency fracture of medial femoral condyle with osseous edema, and (j) a 60-year-old male with mild patellofemoral arthritis.
Table 2 shows the Likert scoring by the two radiologists for different questions in each case. The first question was: “What does the image show?” and the mean score of this question was 2; the second question was: “What is the sequence?” and the mean score of this question was 2.10; the third question was: “What is the key finding?” and the mean score of this question was 1.15; and the last command was: “Write a report of MRI of the……” and the mean score in this was highest, that is, 4.10. The mean score per case by both radiologists ranged from 2.0 to 2.5, and there was no significant difference between different cases when different questions were considered (P > 0.05).
Case no. | Q1: What does the image show? | Q2: What is the sequence? | Q3: What is the key finding? | Q4: Report based on prompt | Mean score per case | ||||
---|---|---|---|---|---|---|---|---|---|
R 1 | R 2 | R 1 | R 2 | R 1 | R 2 | R 1 | R 2 | ||
Figure 1a | 2 | 3 | 2 | 2 | 1 | 1 | 4 | 4 | 2.38 |
Figure 1b | 1 | 1 | 2 | 2 | 1 | 1 | 4 | 4 | 2.00 |
Figure 1c | 2 | 2 | 2 | 2 | 2 | 1 | 5 | 4 | 2.50 |
Figure 1d | 2 | 2 | 2 | 3 | 1 | 1 | 4 | 5 | 2.50 |
Figure 1e | 3 | 3 | 2 | 2 | 1 | 1 | 4 | 4 | 2.50 |
Figure 1f | 2 | 2 | 2 | 2 | 1 | 1 | 4 | 4 | 2.25 |
Figure 1g | 2 | 2 | 2 | 2 | 1 | 1 | 4 | 5 | 2.38 |
Figure 1h | 2 | 2 | 2 | 2 | 1 | 1 | 4 | 3 | 2.13 |
Figure 1i | 3 | 2 | 3 | 2 | 2 | 2 | 4 | 4 | 2.75 |
Figure 1j | 1 | 1 | 2 | 2 | 1 | 1 | 4 | 4 | 2.00 |
Mean score per question | 2.00 | 2.10 | 1.15 | 4.10 |
The agreement assessed by the interclass correlation coefficient between the two raters was 0.92 (95% Confidence interval: 0.85–0.96). The approximate turnaround time for ChatGPT was 10 seconds, and for musculoskeletal radiologists (to analyze and finalize the report), it was 5 min.
DISCUSSION
ChatGPT holds significant potential in contributing to radiology reporting by aiding radiologists and healthcare professionals in the creation of narrative reports, responding to inquiries, and enhancing communication.[6] Here are several prospective applications for utilizing ChatGPT in the context of radiology reporting:
Report generation assistance
ChatGPT aids radiologists in crafting initial reports by translating structured findings into natural language descriptions. Furthermore, it facilitates the condensation of intricate imaging results, enhancing accessibility for both patients and referring physicians. ChatGPT can assist in maintaining consistency across reports by suggesting standardized language and terminology, reducing variations in reporting styles.
Quick reference and information retrieval
Radiologists can use ChatGPT to quickly access reference information, such as guidelines, relevant literature, or case studies, aiding in the interpretation of images and decision-making.
Communication enhancement
ChatGPT can serve as a communication tool between radiologists and other healthcare professionals. It can help clarify technical terms, provide additional context, or answer questions related to radiological findings.
Educational tool
ChatGPT can be used as an educational resource to provide explanations and context for trainees or non-specialists, helping them understand radiological terminology and findings.
Workflow optimization
Integration of ChatGPT into reporting systems can streamline workflows by automating certain aspects of report creation, allowing radiologists to focus more on complex cases and decision-making.
Patient interaction
ChatGPT can be employed to generate patient-friendly summaries of radiology reports, facilitating better communication between healthcare providers and patients. This can improve patient understanding and engagement in their healthcare.[9]
Handling routine queries
ChatGPT can handle routine queries from health-care professionals or administrative staff related to scheduling, report status, or other non-clinical matters, freeing up time for radiologists to focus on their core responsibilities.[6,7]
Incorporating Large Language Models, such as ChatGPT-4V, into the realm of radiological reporting represents a fascinating convergence of AI and medical imaging. Our exploration of the applicability of ChatGPT-4V in analyzing MRI knee images and generating corresponding reports has yielded insightful findings. In our investigation, ChatGPT-4V demonstrated its highest proficiency (rated as good to excellent) when tasked with generating reports based on user-fed clinical information, achieving a mean score of 4.10. Conversely, its performance in tasks involving the interpretation of medical images fell below average (scoring ≤2.10). Specifically, the application faced challenges in identifying the imaging plane in one instance and exhibited inaccuracies in describing key findings across all cases examined. However, it was good in giving recommendations at the end of the report. It is noteworthy that there is currently no published scientific literature assessing the competence of ChatGPT-4 in these particular domains.
Mago and Sharma assessed the potential utility of ChatGPT-3 in oral and maxillofacial radiology, specifically focusing on its application in report writing.[8] The evaluation involved identifying radiographic anatomical landmarks, learning about oral and maxillofacial pathologies, understanding their radiographic features, and assessing ChatGPT-3’s performance and utilization in training for oral and maxillofacial radiology. The study’s findings revealed that ChatGPT-3 is effective in articulating pathology, describing characteristic radiographic features, and outlining anatomical landmarks. While it can serve as a supplementary resource when an oral radiologist requires additional information, it should not be solely relied on as the primary reference. Notably, ChatGPT-3 tends to lack the meticulous attention to detail found in conventional references, posing a risk of information overload and potential medical errors. Despite these limitations, ChatGPT-3 is a valuable tool for enhancing community knowledge and awareness of various pathologies. It plays a role in alleviating patient anxiety by aiding dental healthcare professionals in formulating suitable treatment plans.[8]
ChatGPT or similar language models are not equipped to interpret medical images, including MRI scans of the knee. Analyzing MRI images requires specialized medical knowledge, particularly in the field of musculoskeletal radiology. Radiologists, orthopedic surgeons, or other healthcare professionals with expertise in musculoskeletal imaging are trained to interpret these images accurately. Interpreting MRI images of the knee involves assessing the various structures such as bones, cartilage, ligaments, tendons, and soft tissues. This requires a detailed understanding of normal anatomy as well as the ability to identify abnormalities, injuries, or pathological conditions. While ChatGPT can generate text based on the information provided to it, it is important to note that using a language model for creating medical reports, especially for interpreting MRI images, comes with significant risks and limitations. Generating medical reports requires a deep understanding of radiology, pathology, and clinical context, which AI models like ChatGPT may lack.[10]
CONCLUSION
Interpreting MRI images accurately involves a nuanced understanding of anatomy, pathology, and the ability to correlate findings with a patient’s clinical history. Medical professionals, particularly radiologists, undergo extensive training to develop the necessary expertise for this task. Relying on an AI model for medical report generation may lead to errors, misinterpretations, or incomplete analyses. It is crucial to consult with qualified health-care professionals, such as radiologists or orthopedic specialists, for accurate and reliable interpretation of medical images like MRI scans. These professionals possess the expertise needed to provide a comprehensive analysis based on their medical training and experience.
As ChatGPT continues its ongoing development, the prospect of achieving a successful future model capable of meeting this requirement steadily grows. However, as of the present moment, GPT-4V lacks the capability to interpret medical images and generate accurate reports.
Ethical approval
The Institutional Review Board approval was not required.
Declaration of patient consent
Patient’s consent was not required as there are no patients in this study.
Conflicts of interest
There are no conflicts of interest.
Use of artificial intelligence (AI)-assisted technology for manuscript preparation
The authors confirm that there was no use of artificial intelligence (AI)-assisted technology for assisting in the writing or editing of the manuscript and no images were manipulated using AI.
Financial support and sponsorship
Nil.
References
- A proposal for the dartmouth summer research project on artificial intelligence, August 31, 1955. AI Mag. 2006;27:12.
- [Google Scholar]
- A brief overview of ChatGPT: The history, status quo and potential future development. IEEE/CAA J Automat Sin. 2023;10:1122-36.
- [CrossRef] [Google Scholar]
- Will collaborative publishing with ChatGPT drive academic writing in the future? Br J Surg. 2023;110:1213-4.
- [CrossRef] [PubMed] [Google Scholar]
- Will ChatGPT drive radiology in the future? Indian J Radiol Imaging. 2023;33:436-7.
- [CrossRef] [PubMed] [Google Scholar]
- Revolutionizing radiology with GPT-based models: Current applications, future possibilities and limitations of ChatGPT. Diagn Interv Imaging. 2023;104:269-74.
- [CrossRef] [PubMed] [Google Scholar]
- Radiology gets chatty: The ChatGPT saga unfolds. Cureus. 2023;15:e40135.
- [CrossRef] [Google Scholar]
- The potential usefulness of ChatGPT in oral and maxillofacial radiology. Cureus. 2023;15:e42133.
- [CrossRef] [Google Scholar]
- Enhancing patient communication with Chat-GPT in radiology: Evaluating the efficacy and readability of answers to common imaging-related questions. J Am Coll Radiol. 2024;21:353-9.
- [CrossRef] [PubMed] [Google Scholar]
- ChatGPT and the future of medical writing. Radiology. 2023;307:e223312.
- [CrossRef] [PubMed] [Google Scholar]